|
ACCESS THE FULL ARTICLE
No SPIE Account? Create one
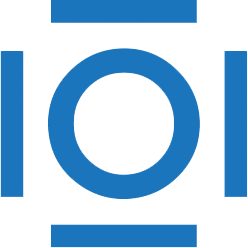
CITATIONS
Cited by 2 scholarly publications.
Databases
Facial recognition systems
Detection and tracking algorithms
Feature extraction
Statistical analysis
Matrices
Principal component analysis