|
ACCESS THE FULL ARTICLE
No SPIE Account? Create one
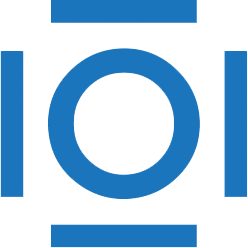
CITATIONS
Cited by 1 scholarly publication.
Image segmentation
Ultrasonography
Signal to noise ratio
Signal attenuation
3D image processing
Expectation maximization algorithms
Breast