|
ACCESS THE FULL ARTICLE
No SPIE Account? Create one
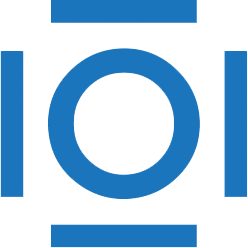
CITATIONS
Cited by 1 scholarly publication.
Associative arrays
Image classification
Reconstruction algorithms
Algorithm development
Data modeling
Image compression
Image processing