Canonical correlation analysis (CCA) is a popular method that has been extensively used in feature learning. In nature, the objective function of CCA is equivalent to minimizing the distance of the paired data, and L2-norm is used as the distance metric. We know that L2-norm-based objective function will emphasize the large distance pairs and de-emphasizes the small distance pairs. To alleviate the aforementioned problems of CCA, we propose an approach named CCA based on L1-norm minimization (CCA-L1) for feature learning. To optimize the objective function, we develop an algorithm that can get a global optimized value. To maintain the distribution and the nonlinear characteristic respectively, we proposed two extensions of CCA-L1. Further, all of the aforementioned three proposed algorithms are extended to deal with multifeature data. The experimental results on an artificial dataset, real-world crop leaf disease dataset, ORL face dataset, and PIE face dataset show that our methods outperform traditional CCA and its variants. |
ACCESS THE FULL ARTICLE
No SPIE Account? Create one
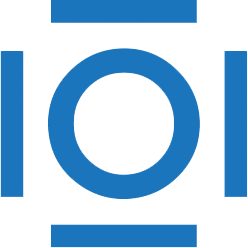
CITATIONS
Cited by 3 scholarly publications.
Simulation of CCA and DLA aggregates
Canonical correlation analysis
Algorithm development
Feature extraction
Convex optimization
Detection and tracking algorithms
Distance measurement