Recently, deep neural networks have made remarkable performance in the image super-resolution (SR) field. However, they mainly focus on wider or deeper architectural design, neglecting to capture the inherent property of natural images, hence hindering the representational ability of convolutional neural networks. To address this issue, we propose a deep network based on nonlocal (NL) and second-order (SO) feature fusion for image SR. In particular, we draw the observation that a SO attention mechanism could achieve more powerful feature expression and feature correlation learning. On the other hand, NL module is proved to be an effective prior to explore spatial contextual information. Thus, we introduce an SR network architecture by embedding NL operations and SO feature to capture intrinsic statistical characteristics of images. Furthermore, long skip connection is applied in the network to pass more abundant low-frequency information from low-resolution images and ease the network training. Experimental results on a variety of images demonstrate that our proposed method can achieve more desirable performance over several state-of-the-art methods in terms of quantitative metrics and visual quality. |
ACCESS THE FULL ARTICLE
No SPIE Account? Create one
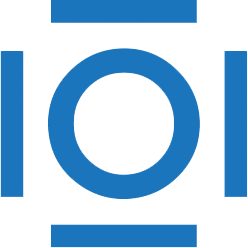
CITATIONS
Cited by 3 scholarly publications.
Image fusion
Super resolution
Convolution
Lawrencium
Visualization
Image restoration
Feature extraction