ACCESS THE FULL ARTICLE
No SPIE Account? Create one
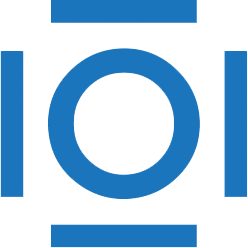
CITATIONS
Cited by 1 scholarly publication.
Education and training
Cancer
Tissues
Lung
Tumors
Performance modeling
Deep learning