ACCESS THE FULL ARTICLE
No SPIE Account? Create one
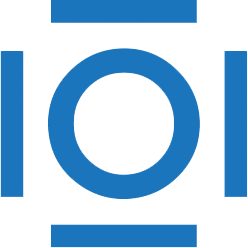
CITATIONS
Cited by 1 scholarly publication.
Kidney
Image segmentation
Magnetic resonance imaging
Cancer detection
Data modeling
Solids
Education and training