|
ACCESS THE FULL ARTICLE
No SPIE Account? Create one
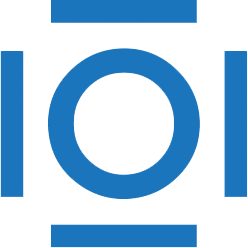
CITATIONS
Cited by 145 scholarly publications and 4 patents.
Breast
Tissues
Image classification
Convolutional neural networks
Classification systems
Cancer
Diagnostics