|
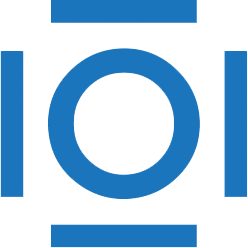
CITATIONS
Cited by 49 scholarly publications and 2 patents.
Skin
Image segmentation
Data modeling
Network architectures
Image filtering
Melanoma
Performance modeling