Purpose: Deep learning (DL) algorithms have shown promising results for brain tumor segmentation in MRI. However, validation is required prior to routine clinical use. We report the first randomized and blinded comparison of DL and trained technician segmentations. Approach: We compiled a multi-institutional database of 741 pretreatment MRI exams. Each contained a postcontrast T1-weighted exam, a T2-weighted fluid-attenuated inversion recovery exam, and at least one technician-derived tumor segmentation. The database included 729 unique patients (470 males and 259 females). Of these exams, 641 were used for training the DL system, and 100 were reserved for testing. We developed a platform to enable qualitative, blinded, controlled assessment of lesion segmentations made by technicians and the DL method. On this platform, 20 neuroradiologists performed 400 side-by-side comparisons of segmentations on 100 test cases. They scored each segmentation between 0 (poor) and 10 (perfect). Agreement between segmentations from technicians and the DL method was also evaluated quantitatively using the Dice coefficient, which produces values between 0 (no overlap) and 1 (perfect overlap). Results: The neuroradiologists gave technician and DL segmentations mean scores of 6.97 and 7.31, respectively (p < 0.00007). The DL method achieved a mean Dice coefficient of 0.87 on the test cases. Conclusions: This was the first objective comparison of automated and human segmentation using a blinded controlled assessment study. Our DL system learned to outperform its “human teachers” and produced output that was better, on average, than its training data. |
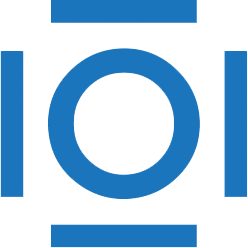
CITATIONS
Cited by 9 scholarly publications.
Image segmentation
Tumors
Brain
Magnetic resonance imaging
Neural networks
Cancer
Neuroimaging