Purpose: We investigate the impact of various deep-learning-based methods for detecting and segmenting metastases with different lesion volume sizes on 3D brain MR images. Approach: A 2.5D U-Net and a 3D U-Net were selected. We also evaluated weak learner fusion of the prediction features generated by the 2.5D and the 3D networks. A 3D fully convolutional one-stage (FCOS) detector was selected as a representative of bounding-box regression-based detection methods. A total of 422 3D post-contrast T1-weighted scans from patients with brain metastases were used. Performances were analyzed based on lesion volume, total metastatic volume per patient, and number of lesions per patient. Results: The performance of detection of the 2.5D and 3D U-Net methods had recall of >0.83 and precision of >0.44 for lesion volume >0.3 cm3 but deteriorated as metastasis size decreased below 0.3 cm3 to 0.58 to 0.74 in recall and 0.16 to 0.25 in precision. Compared the two U-Nets for detection capability, high precision was achieved by the 2.5D network, but high recall was achieved by the 3D network for all lesion sizes. The weak learner fusion achieved a balanced performance between the 2.5D and 3D U-Nets; particularly, it increased precision to 0.83 for lesion volumes of 0.1 to 0.3 cm3 but decreased recall to 0.59. The 3D FCOS detector did not outperform the U-Net methods in detecting either the small or large metastases presumably because of the limited data size. Conclusions: Our study provides the performances of four deep learning methods in relationship to lesion size, total metastasis volume, and number of lesions per patient, providing insight into further development of the deep learning networks. |
ACCESS THE FULL ARTICLE
No SPIE Account? Create one
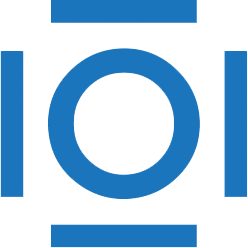
CITATIONS
Cited by 9 scholarly publications.
Brain
Image segmentation
3D image processing
Neuroimaging
Magnetic resonance imaging
3D modeling
Image fusion