Purpose: We developed a deep learning method to reduce noise and beam-hardening artifact in virtual monoenergetic image (VMI) at low x-ray energy levels. Approach: An encoder–decoder type convolutional neural network was implemented with customized inception modules and in-house-designed training loss (denoted as Incept-net), to directly estimate VMI from multi-energy CT images. Images of an abdomen-sized water phantom with varying insert materials were acquired from a research photon-counting-detector CT. The Incept-net was trained with image patches (64 × 64 pixels) extracted from the phantom data, as well as synthesized, random-shaped numerical insert materials. The whole CT images (512 × 512 pixels) with the remaining real insert materials that were unseen in network training were used for testing. Seven contrast-enhanced abdominal CT exams were used for preliminary evaluation of Incept-net generalizability over anatomical background. Mean absolute percentage error (MAPE) was used to evaluate CT number accuracy. Results: Compared to commercial VMI software, Incept-net largely suppressed beam-hardening artifact and reduced noise (53%) in phantom study. Incept-net presented comparable CT number accuracy at higher-density (P-value [0.0625, 0.999]) and improved it at lower-density inserts (P-value = 0.0313) with overall MAPE: Incept-net [2.9%, 4.6%]; commercial-VMI [6.7%, 10.9%]. In patient images, Incept-net suppressed beam-hardening artifact and reduced noise (up to 50%, P-value = 0.0156). Conclusion: In this preliminary study, Incept-net presented the potential to improve low-energy VMI quality. |
ACCESS THE FULL ARTICLE
No SPIE Account? Create one
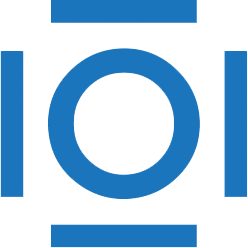
CITATIONS
Cited by 7 scholarly publications and 1 patent.
X-ray computed tomography
Computed tomography
Denoising
Iodine
Image quality
Data acquisition
Image processing