Purpose: Multiparametric magnetic resonance imaging (mp-MRI) is being investigated for kidney cancer because of better soft tissue contrast ability. The necessity of manual labels makes the development of supervised kidney segmentation algorithms challenging for each mp-MRI protocol. Here, we developed a transfer learning-based approach to improve kidney segmentation on a small dataset of five other mp-MRI sequences. Approach: We proposed a fully automated two-dimensional (2D) attention U-Net model for kidney segmentation on T1 weighted-nephrographic phase contrast enhanced (CE)-MRI (T1W-NG) dataset (N = 108). The pretrained weights of T1W-NG kidney segmentation model transferred to five other distinct mp-MRI sequences model (T2W, T1W-in-phase (T1W-IP), T1W-out-of-phase (T1W-OP), T1W precontrast (T1W-PRE), and T1W-corticomedullary-CE (T1W-CM), N = 50) and fine-tuned by unfreezing the layers. The individual model performances were evaluated with and without transfer-learning fivefold cross-validation on average Dice similarity coefficient (DSC), absolute volume difference, Hausdorff distance (HD), and center-of-mass distance (CD) between algorithm generated and manually segmented kidneys. Results: The developed 2D attention U-Net model for T1W-NG produced kidney segmentation DSC of 89.34 ± 5.31 % . Compared with randomly initialized weight models, the transfer learning-based models of five mp-MRI sequences showed average increase of 2.96% in DSC of kidney segmentation (p = 0.001 to 0.006). Specifically, the transfer-learning approach increased average DSC on T2W from 87.19% to 89.90%, T1W-IP from 83.64% to 85.42%, T1W-OP from 79.35% to 83.66%, T1W-PRE from 82.05% to 85.94%, and T1W-CM from 85.65% to 87.64%. Conclusions: We demonstrate that a pretrained model for automated kidney segmentation of one mp-MRI sequence improved automated kidney segmentation on five other additional sequences. |
ACCESS THE FULL ARTICLE
No SPIE Account? Create one
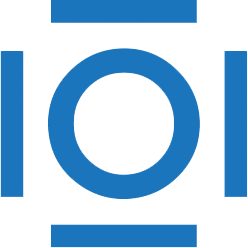
CITATIONS
Cited by 2 scholarly publications.
Kidney
Image segmentation
Data modeling
Magnetic resonance imaging
3D modeling
Performance modeling
Statistical modeling