1.IntroductionThe ability to monitor tissue oxygen saturation () non-invasively by near-infrared spectroscopy (NIRS) has garnered considerable interest in clinical scenarios associated with a risk of brain damage, including certain surgical procedures and during intensive care.1 More recently, the combination of NIRS with diffuse correlation spectroscopy (DCS)2 offers the opportunity to simultaneously measure multiple cerebral hemodynamic and metabolic parameters, including cerebral blood flow (CBF), blood volume, and markers of oxygen metabolism.3–7 In addition to clinical bedside monitoring,4,8–11 both DCS and NIRS have the temporal resolution to measure transient changes in cerebral hemodynamics in response to various physiological paradigms designed to assess the mechanisms regulating CBF. These paradigms include hypercapnia to assess chemoregulation,3 head-up tilt,12 and thigh-cuff release13,14 to assess autoregulation related to vessel compliance and myogenic tone, and functional activation to assess neuronal control.15 Traditionally, non-invasive cerebral autoregulation has been assessed using transcranial Doppler ultrasound (TCD) to track changes in middle cerebral artery blood velocity (MCAv). While TCD is also non-invasive, it requires a constant and precise location of the ultrasound probe and cannot be used for long-term monitoring. Furthermore, since TCD measures blood velocity and not flow, it relies on the assumption that vascular tone remains stable throughout the assessment.16 In contrast, the combination of DCS and NIRS offers the ability to measure blood flow regulation at the microvascular level, and the combination of microvascular CBF and provides a means of assessing the potential impact of altered flow regulation on oxygen delivery and energy metabolism in the brain. Despite the potential of these non-invasive optical techniques to study cerebrovascular regulation, interpreting NIRS and DCS data can be confounded by signal contamination from extracerebral tissue since the depth to the brain in the adult head varies between 1 and 2 cm.17–19 Consequently, over 80% of the signal at source-detector distance of originates from hemodynamic changes in the scalp, easily overshadowing the cerebral component and resulting in substantial errors in and CBF measurements.19,20 Several approaches have been developed to reduce the effects of scalp contamination on NIRS and DCS, with the most common being to acquire data at multiple source-detector distances ().21–23 More advanced technologies, i.e., time-resolved and frequency-domain methods, have proven effective for enhancing the depth sensitivity of NIRS.24–27 Time-resolved detection has also been applied to DCS28; however, this approach is challenging due to the poorer signal-to-noise ratio of current DCS technology.29 Consequently, multi-distance continuous-wave DCS remains the most commonly used approach in neuromonitoring applications. The substantially higher blood flow in the brain compared to the scalp gives DCS an inherent advantage in terms of depth sensitivity compared to NIRS.30 The importance of incorporating methods to separate cerebral and extracerebral signal contributions is particularly relevant to physiological stimuli considering many have systemic effects that likely alter scalp blood flow. For example, by collecting time-resolved NIRS (trNIRS) data at short and long source-detector distances ( and 3 cm), Milej et al.31,32 demonstrated different vasoreactivity in scalp and brain in response to hypercapnia. If DCS and NIRS are to be used reliably in clinical applications involving bedside neuromonitoring, it is critical to understand the impact of extracerebral signal contributions on CBF and measurements during physiological perturbations, such as by changes in posture. The purpose of this study was to investigate signal contamination from changes in scalp blood flow during transient hypotension.13 Measuring the CBF response to changes in blood pressure is a well-established method for characterizing cerebral autoregulation.33 Therefore, it is critical to evaluate the magnitude of extracerebral signal contamination in NIRS and DCS data and assess the suitability of technique-relevant methods for separating scalp and brain signals. For this study, a hybrid trNIRS/multidistance DCS system was used to acquire oxygenation and blood flow data during a rapid-onset lower body negative pressure (LBNP) challenge. This paradigm is a commonly used technique to mimic orthostatic stress (i.e., standing up) while the participant remains in a supine posture and is frequently used to assess cerebral autoregulation with TCD.34 Analysis of multidistance DCS data was based on the modified Beer-Lambert approach proposed by Baker et al.35 This approach requires applying pressure to the scalp to evaluate the sensitivity of DCS probes at different source-detector distances to scalp blood flow. In addition, a pneumatic tourniquet wrapped around the head was used to reduce scalp blood flow. The LBNP experiment was repeated with the tourniquet fully inflated to temporarily restrict scalp blood flow, thereby isolating the CBF response.31 Similarly, the influence of changes in scalp oxygenation on trNIRS measurements of and cerebral blood volume during LBNP was investigated. Time gates and moment analysis were applied to the trNIRS data to extract signals weighted to the superficial tissue (i.e., early-arriving photons) and brain (i.e., late-arriving photons). Finally, TCD was used to measure changes in MCAv in response to LBNP for comparison to the microvascular CBF responses measured by DCS. 2.Methods2.1.InstrumentationAll data were collected using an in-house built trNIRS/DCS system.3,36 For the DCS module, light from a continuous laser with a long coherence length () emitting at (DL852-100-SO, CrystaLaser) was coupled into a single fiber (fiber core diameter , , Loptek, Germany). On the detection side, reflected light was collected by one single-mode fiber at a source-detector separation () of 1 cm and three fibers at (core diameter , , Loptek, Berlin, Germany). Detection fibers were coupled to a four-channel single-photon counting module (SPCMAQR-15-FC, Excelitas Technologies, Canada). Each counting module generated TTL pulses sent to an edge-detecting photon counter on a PCIe6612 counter/timer data acquisition board (National Instrument).37 Photon counts were recorded and processed using in-house developed software written in LabVIEW (2019, National Instruments) and MATLAB (2017, MathWorks). Intensity autocorrelation curves were generated for 50 delay times () ranging from to 1 ms. The trNIRS module included two pulsed laser heads emitting at and 830 nm, controlled by a Sepia II laser driver operating at 80 MHz (PicoQuant, Germany). The laser heads were coupled to multimode bifurcated fiber (core diameter , NA = 0.39, Loptek, Germany) to deliver the light to the scalp. A detection fiber bundle (3.6 mm, NA = 0.55, Loptek, Germany) collected diffusively reflected light from the scalp at and was coupled to a hybrid photomultiplier tube (PMA Hybrid 50, PicoQuant, Germany).38 A time-correlated single-photon counting module (HydraHarp 400, PicoQuant, Germany) was used to record photon arrival times and generate a time-of-flight distribution of diffusely reflected photons (DTOF).32,39 To acquire trNIRS/DCS data simultaneously, a short-pass interference filter was placed between collimating lenses in front of trNIRS detector (Spec 3551, 836.5 nm, diameter 25 mm, Alluxa, Santa Rosa, California, United States).3 At the end of every study, the instrument response function (IRF) was measured using a custom-built light-tight box that connected the emission fiber to a detection probe with a separation of 10 cm. 2.2.Experimental ProcedureAll procedures were approved by the Health Sciences Research Ethics Board at Western University (Grant No. 120391) and Lawson Health Research Institute (Grant No. 107985), adhering to the guidelines of the Tri-Council Policy Statement for research involving humans. Participants provided written informed consent following verbal and written explanations of the experimental procedures. Ten healthy participants (5 women, years, , ) with no history of any neurological or psychiatric disorders were recruited. All participants identified with the sex that was assigned to them at birth. Participants completed two protocols, one with the tourniquet [Fig. 1(a)] off and the other with the tourniquet on [Fig. 1(b)]. To create a rapid but brief (15 s) bout of orthostatic stress, participants were sealed from the waist down in an LBNP chamber. The cerebral hemodynamic responses were assessed for a short (15 s) pulse of rapid-onset LBNP with suction levels of . For this protocol, cerebrovascular hemodynamics were assessed with DCS, trNIRS, and TCD. All three were acquired continuously throughout the LBNP protocol at a sampling rate of 3.33 Hz for trNIRS/DCS and 3.33 Hz for TCD. Fig. 1(a) Illustration of optical probes placement and (b) experimental paradigms used in the study. Light sources and detectors on the probe holder are color-coded. Red, NIRS source; pink, DCS source; dark blue, DCS detector (); light blue, DCS detector (); green, trNIRS detector (). ![]() The DCS and trNIRS probes were secured to the forehead using a custom 3D-printed holder made of flexible resin (Flexible 80A, Formlabs, Somerville, Massachusetts, United States). Confounding effects related to placing probes close to the sagittal sinus were considered; however, Liu et al.40 showed that NIRS measurements are primarily sensitive to oxygenation in the microvasculature as almost all light that interrogates larger vessels, such as the sagittal sinus, is absorbed. Placing the probes on the midline may have resulted in a greater loss of light, but the signals from both trNIRS and DCS were found to be adequate for this study. A finger photoplethysmography was used to provide continuous arterial blood pressure (ABP) recordings during LBNP (Finometer, Finapres Medical Systems, Enschede, The Netherlands). Mean ABP (MAP) was calculated using the algebraic mean for each cardiac cycle. The finger unit was calibrated against three manual sphygmomanometric brachial artery measures. Expired gas was sampled at the mouth to measure partial pressure end-tidal (; ML206 analyser). Finally, participants were instrumented with TCD (Neurovision TOC2M, Multigon Industries, Elmsford, New York, United States) for measures of right MCAv (M1 segment). The ultrasound probe was held in place with a headband device. As a means of assessing scalp blood flow contributions to the DCS signal, the LBNP experiment was repeated after inflating a 10-cm wide tourniquet wrapped around the head, just above the supraorbital ridge.31 The tourniquet was designed to impede blood flow to the scalp by inflating two bladders positioned over the temples. The tourniquet included an opening for the optical probe holder () that was large enough to ensure the tourniquet did not press down on the holder when inflated [Fig. 1(a)]. DCS data were acquired continuously while inflating the tourniquet to 170 or 50 mmHg above systolic blood pressure for 120 s. The tourniquet remained at the higher pressure while the LBNP protocol was repeated [Fig. 1(b)]. 2.3.Data Analysis2.3.1.trNIRSTo determine baseline optical properties for each subject, a mean DTOF was generated from the first minute of baseline recordings (i.e., prior to LBNP) for each wavelength. Each DTOF was fit with the solution to the diffusion equation for a semi-infinite homogeneous medium convolved with the measured IRF (fminsearch, MATLAB, MathWorks Inc., Natick, Massachusetts, United States). The fitting parameters were the baseline absorption coefficient (), reduced scattering coefficient () and an amplitude factor that accounts for laser power, detection gain, and coupling efficiency. The fitting range was set to 10% of the peak value of a DTOF on the leading edge and 1% on the falling edge. Next, each DTOF in a time series recorded at 760 and 830 nm was analyzed to calculate the first three statistical moments: the number of photons (), the mean time of flight (), and the variance (). The moments were calculated by setting the lower and upper integration limits based on arrival times corresponding to 1% of the peak of the DTOF.41,42 The change in each moment relative to its initial baseline value was calculated to generate three time series (i.e., , , and ) for the two wavelengths individually. Time courses for each statistical moment were converted into absorption changes () using sensitivity analysis as described in detail elsewhere.32,43 The sensitivity factors were generated using subject-specific baseline optical properties obtained through the fitting routine described above (mean and at 760 nm; mean and at 830 nm). The time courses recorded at 760 and 830 nm were converted to changes in concentration of oxyhemoglobin () and deoxyhemoglobin () using their respective molar extinction coefficients.44 and time courses were used to determine the corresponding change in tissue oxygen saturation () using the standard definition of : . The sum of and was also used to determine changes in the total hemoglobin concentration (ΔtHb), which reflects changes in blood volume, assuming no change in hemoglobin concentration. In addition to moment analysis, two gates, one early and one late, were extracted from each DTOF to generate time-varying changes in attenuation () with sensitivity to the scalp and cerebral oxygenation, respectively.32 Both gates had a width of 250 ps.45 The start of the early gate was positioned at the rising edge of the DTOF when the signal intensity reached 1% of the peak value, whereas the end of the late gate was located on the opposite DTOF edge where the intensity dropped to 1%. Analogous to moment analysis, time series for the two gates measured at the two wavelengths were converted to , using corresponding sensitivity factors.32 Similar to the statistical moment analysis described above, time courses of and were used to calculate and . All time courses were smoothed with a 0.9-s (three data points) moving average with a zero-phase digital filter (filtfilt, MATLAB, MathWorks Inc., Natick, Massachusetts, United States). 2.3.2.DCS—semi-infinite modelNormalized intensity autocorrelations functions were converted to electric field autocorrelation data using the Siegert relation.46 Each autocorrelation function was subsequently fit with the solution to the diffusion approximation for a semi-infinite homogenous medium to estimate the blood flow index (BFi), assuming tissue perfusion is modeled as a pseudo-Brownian motion.2 The fitting incorporated each subject’s and values measured by trNIRS. Fitting was performed across all correlation times from to 1 ms. The resulting BFi time courses were smoothed with the same filter (zero-phase digital filtering, filtfilt, MATLAB, 2016b, MathWorks, Natick, Massachusetts, United States) as the trNIRS data. 2.3.3.DCS—multilayered modelDepth-enhanced DCS analysis was based on the probe pressure modulation algorithm for CBF monitoring described by Baker et al.35 Based on a two-layer modified Beer-Lambert approach, changes in CBF during LBNP were extracted from the multidistance DCS data using where, , represents the sensitivity to the brain layer for DCS data recorded at . and are the changes in the DCS optical density measured at the short (1 cm) and long (2.5 cm) distances, respectively. and are the changes in optical density measured in response to a change in scalp blood flow. In these experiments, and were obtained from inflating the head tourniquet. A change in optical density at a given source-detector distance is defined by where, is the measured intensity autocorrelation function.Baseline blood flow indices for scalp and brain ( and , respectively) were estimated using a three-layered DCS model that represents blood flow in scalp, skull and brain.23 The model was applied to intensity autocorrelation data acquired at before and after inflating the tourniquet. Analysis was performed assuming did not change between the two conditions and skull blood flow was set to 1% of since bone blood flow is expected to be low.47 was assumed to be the same in all three layers and set to the value measured by trNIRS for each subject. Similarly, for the brain and scalp was set to subject-specific values from trNIRS, and for the skull was set equal to 60% of based on Strangman et al.48 The thicknesses of the scalp and skull layers were set to average values from a previous study: 0.65 cm for scalp and 0.55 cm for the skull.36 The final step was to estimate , which was obtained from the three-layered model for a 1% change in CBF and using subject-specific values of and . For the data obtained for a fully inflated (170 mmHg) head tourniquet, the change in optical density at measured for each subject was scaled by subject-specific and . Since the distance to the brain () was not measured for each subject, the analysis described above was performed for values from 1 to 1.4 cm, assuming a fixed ratio of the scalp to skull thicknesses of 1.2. 2.3.4.Numerical simulationsFor comparison to the experiment data, the three-layered model was used to generate simulated data at and 2.5 cm over a range of values from 0.9 to 1.4 cm and using average values of and . Simulated data were generated for a reduction in scalp blood flow of 85% to reflect the effect of the tourniquet and for comparison an 85% reduction in CBF. Data at the two source-detector distances were analyzed individually using the semi-infinite model to predict the expected change in BFi. 2.4.Statistical AnalysisAll data are presented as mean ± standard deviation unless otherwise noted. Statistical significance was defined as . Paired -tests were used to assess the effect of LBNP on each variable (i.e., the last 5 s of LBNP compared to the 10-s baseline). For DCS variables, an additional paired -test was used to assess the difference between CBF calculations derived from the multilayer model with and without the tourniquet inflation. A two-way analysis of variance (ANOVA) of time (two levels: baseline, nadir) and (two levels: 1 cm, 2.5 cm) evaluated the BFi response to LBNP between long and short . For trNIRS, paired -tests were used to evaluate differences between variance and both early or late gates and the early gate compared to the number of photons. Changes in MCAv were assessed using one-way repeated measures ANOVA with a fixed factor of time (10 levels: 3 s bins across 30 s) for LBNP trials at . 3.ResultsOf the 10 participants, 1 was omitted due to large intensity fluctuations ( of the curve mean) in the autocorrelation curves and low photon count () in the DTOFs, which were attributed to poor skin-to-probe contact. All participants tolerated the LBNP and tourniquet inflation. Figure 2(a) illustrates relative BFi (rBFi) changes measured at the two source-detector distances in response to inflating the head tourniquet to 170 mmHg. The rBFi reduction was greater at than 2.5 cm for 7 out of 9 participants (all ), with an average difference of (). For the remaining two participants, the measured rBFi reductions at were within the range found for the other participants. The smaller responses measured at may have been due to unequal pressure around the probes when the tourniquet was inflated. Due to this unexpected experimental issue, these two data sets were excluded from the calculation of using Eq. (1). Fig. 2(a) Measured change in rBFi for each subject in response to inflating the tourniquet to 170 mmHg. (b) Predicted change in rBFi at two source-detectors distances (, squares and 2.5 cm, circles) as a function of distance to the brain. Results are presented for an 85% reduction in scalp blood flow (black lines) and an 85% reduction in CBF (red lines). ![]() The numerical simulations [Fig. 2(b)] predicted a BFi decrease of 77% at and 83% at when the distance to the brain is 1.2 cm, which is the average distance on the forehead measured previously by magnetic resonance imaging (MRI).36 This prediction in good agreement with the experimental results. Of note, a change in CBF at the same distance is predicted to cause approximately a 20% change in the BFi measured at and only 1.5% at . LBNP of produced a significant decrease in MAP of [; ; Fig. 3(a)]. The average change in MAP during LBNP after tourniquet inflation was not statistically different (; ). End-tidal decreased slightly, albeit not significant during LBNP ( to , ). Corresponding time-varying changes in rBFi recorded at the two source-detector distances are presented in Fig. 3(b). Both distances showed a significant reduction in rBFi ( versus baseline). The decrease calculated for the last 5 s of LBNP was for and for . No statistical difference was observed in the responses obtained at the two source-detector separations ( interaction and the main effect of : ). Fig. 3Average changes in (a) MAP and (b) rBFi plotted as a function of time during LBNP, which is indicated by the grey region between 10 and 25 s. DCS data were recorded at and 2.5 cm without inflating the head tourniquet. Shadowing surrounding each time course represents the standard deviation across subjects. ![]() Time-varying changes in the NIRS parameters (i.e., , , , and ΔtHb) during the LBNP challenge are presented in Fig. 4. These time courses were generated from the metrics with the poorest depth sensitivity, namely from the early gate and . For both measurements, decreases in during LBNP were statistically significant (both versus baseline). No significant difference was found between obtained for the early gate and (). LBNP did not drive a significant change in , or for or (all versus baseline). Fig. 4Average , , and responses to LBNP (between 10 and 25 s). Time courses are presented for the change in attenuation measured in an early time gate and from the total number of photons (). All time courses were averaged across nine subjects. Shadowing represents the standard deviation across subjects. ![]() , , , and time courses derived from the two metrics with the highest depth sensitivity (i.e., from a late gate and ) are presented in Fig. 5. No significant change occurred during LBNP for any variable obtained by the late gate and analyses (all ). However, changes in obtained from the variance and late-gate were significantly smaller than the corresponding changes obtained from the early-gate analysis (). Fig. 5Average , , , and responses to LBNP (between 10 and 25 s). Time courses are presented for metrics with greater depth sensitivity (i.e., from the late gate and the change in variance, ). All time courses were averaged across nine subjects. Shadowing represents the standard deviation across subjects. ![]() Figure 6 presents representative autocorrelation curves obtained at the baseline () and increased tourniquet pressure () measured at the two source-detector separations and the corresponding ratio of . For each subject, was calculated for values from 10 to , as this range avoided instabilities evident at shorter and longer delay times.35 Table 1 provides subject-specific baseline optical properties obtained with trNIRS, measurements, and estimates of , and from the three-layered DCS model. Note, values are not presented for the two subjects with the unexpected tourniquet response at the short . Fig. 6Representative autocorrelation curves (one subject) for the two source-detector separations (left) measured at baseline (superscript “0”) and after inflating the tourniquet (superscript “P”). Corresponding ratio as a function of correlation time is shown on the right. Dashed red lines represent a range of delay times selected to calculate the average ratio. ![]() Table 1Subject-specific estimates of baseline optical properties measured by trNIRS, ΔODLP/ΔODSP, baseline blood flows (FS,0, FC,0), and the cerebral sensitivity factor obtained from the three-layered DCS model.
Figure 7(a) presents average time courses of the microvascular CBF response to LBNP across the seven subjects. Time courses are shown for DCS data acquired with or without the tourniquet inflated. For each subject, the CBF response derived using Eq. (1) was scaled by a subject-specific , from the three-layered model. The reconstructed changes in CBF during LBNP [Fig. 7(a)] were significantly smaller than the BFi values obtained using the semi-infinite model (Fig. 3; ) and did not show a significant change during LBNP (both versus baseline). Further, the reconstructed blood flow changes () and the blood flow response measured after the tourniquet was inflated () were not significantly different from each other (). For comparison, the average MCAv response to LBNP is shown in Fig. 7(b). The MCAv response to LBNP was not significant across time. At the nadir, the average reduction was (one-way ANOVA: ). Fig. 7(a) Average relative CBF (rCBF) response to the LBNP challenge between 10 to 25 s. Time courses are shown for DCS data acquired with and without the tourniquet inflated. (b) Mean MCAv () during LBNP. TCD data were acquired simultaneous to DCS (the tourniquet off condition). Shadowing represents the standard deviation across seven subjects. ![]() 4.DiscussionThis study utilized a hybrid trNIRS/multidistance DCS system to assess the magnitude of extracerebral signal contamination in NIRS and DCS data acquired during transient hypotension (induced by LBNP) and the suitability of technique-relevant methods for separating scalp and brain measurements. The motivation was to improve the confidence when applying these non-invasive optical technologies to applications in critical-care settings, given the clinical interest in using flow and metabolic markers to assess brain health and prevent secondary brain injury. The primary outcome was demonstrating that transient hypotension caused significantly larger blood flow and oxygenation changes in the extracerebral tissue compared to the brain. Further, depth-enhanced methods proved effective at removing this signal contamination. The impact of extracerebral signal contamination on DCS blood flow measurements was evaluated by acquiring data at two source-detector distances. A large (i.e., ), statistically significant decrease in blood flow was detected when data acquired at the two distances were analyzed separately using the semi-infinite model, which neglects the layered structure of the head. There was no significant difference in the magnitude of the blood flow responses measured at the two distances; moreover, the two time courses were extremely similar, as reflected by the non-significant interaction (Fig. 3). These results suggest a common signal contribution, which was likely the scalp considering the limited brain sensitivity for the detector at [Fig. 2(b)]. Similar decreases in blood flow measured by DCS at a single source-detector distance have also been reported in response to thigh cuff deflation, which causes a comparable MAP reduction to the rapid-onset LBNP used in the current study.13,14 Further evidence of a substantial signal contribution from the scalp was the large reduction in the DCS BFi measured at both distances when the head tourniquet was inflated (). These results indicate that the DCS data acquired at are susceptible to extracerebral signal contamination and should not be treated solely as a marker of CBF. To isolate the CBF response to LBNP, the multidistance DCS data were analyzed using a two-layer model that incorporated a pressure modulation procedure aimed at assessing the sensitivity of the two distances to scalp blood flow.35 The large contrast between the reconstructed CBF time course obtained from the model (Fig. 7) and the blood flow changes measured at the two distances individually (Fig. 3) demonstrates the importance of using multi-layered models to separate scalp and brain signal contributions. Similar approaches have been used to isolate CBF responses to thigh cuff-induced transient hypotension14 and to hypercapnia.31 To confirm the CBF time course derived from the model, the LBNP experiment was repeated after inflating a pneumatic tourniquet to impede scalp blood flow. In agreement with the modeling results, the measured blood flow response showed no significant decrease during LBNP (Fig. 7). Similarly, no significant reduction in MCAv as measured by TCD was found (Fig. 7). The small discrepancy between the average reductions in MCAv and DCS-CBF could be explained by the error in the DCS analysis introduced by assuming an average scalp/skull thickness of 1.2 mm. To investigate the impact of this assumption, the analysis of the LBNP data was repeated while varying the distance to the brain from 1 to 1.4 cm. The corresponding CBF reductions ranged from to , none of which were significantly different from baseline (all ), indicating that the cerebral microvasculature had minimal response to LBNP. The discrepancy between MCAv and DCS, despite neither being significant, may have been due to increased vascular compliance, designed to protect the microcirculation from hypoperfusion. The considerable differences in blood flow response in brain and scalp to LBNP illustrate the expected differences in flow regulation in central and peripheral tissues. Myogenic, metabolic, endothelial, and neurogenic mechanisms are involved in the maintenance of CBF during fluctuations in blood pressure. However, the degree of flow regulation is not as critical to scalp tissue considering the low metabolic demands of resting skin and muscle and its ability to store oxygen in myoglobin. The contrasting responses between scalp and brain have also been observed with respect to vascular reactivity to hypercapnia. While it is well known that the cerebral vasculature is exquisitely sensitive to changes in arterial tension,49 vascular reactivity in the scalp is considerably muted and slower by comparison.3 Considering that blood pressure management is a central component of critical care, differences in vascular regulation between brain and scalp again highlight the need for multi-layer modeling approaches for disentangling flow changes in the two tissues. The lack of cerebral response to the rapid-onset LNBP was also observed in the trNIRS results. Although multidistance measurements can be combined with trNIRS to improve the separation of the scalp and cerebral components, a time gate positioned at the rising edge of a DTOF recorded at a distance typically used for neuromonitoring () will be predominately sensitive to extracerebral tissue.32 Likewise, a time gate placed at the trailing edge of a DTOF or a higher moment will provide greater sensitivity to the brain. Using the depth-sensitivity built into time-of-flight data, this study demonstrated that oxygenation changes sensitive to superficial tissue were significantly reduced during LBNP (Fig. 4). Reduced during LBNP has been reported in previous studies using commercial NIRS systems.50,51 However, scalp contamination could not be ruled out as also correlated with skin blood flow measured by laser Doppler flowmetry during LBNP.52 In the current study, none of the oxygenation metrics derived from either the late gate or variance exhibited a significant change in response to LBNP (Fig. 5). Furthermore, changes obtained from these two depth-sensitive methods were significantly smaller than the changes derived from the early gate and analyses, reflecting the differences in scalp and brain vascular responses to a sudden drop in blood pressure. The total hemoglobin concentration was used as a marker of relative blood volume changes, and the negligible decrease during LBNP (Fig. 5) confirms the CBF results obtained from the multidistance DCS data. A limitation of deriving CBF from a multi-layer model is the increase in the number of variables, including the optical properties of the different tissue layers and the thicknesses of the extracerebral layers.23 While trNIRS provided estimates of baseline optical properties in the current study, estimates of scalp and skull thickness were based on previously MRI measurements. As discussed above, calculating the rCBF response to LBNP using a range of skull/scalp thicknesses did not substantially change the time course shown in Fig. 7. However, the uncertainty introduced using an assumed value likely contributed to high inter-subject variability observed in and (Table 1).53 In comparison, inter-subject variability in baseline CBF is typically .36 It is noteworthy that the two participants with the largest discrepancies in baseline (numbers 3 and 8) and highest baseline values ( and , respectively) contributed the most to the intersubject variability in . Removing these two lowered the variability from to 23%. Note that the outcomes of the LBNP experiment were the same with and without including these participants. Incorporating the extracerebral thickness as an additional fitting parameter in the optimization routine has been suggested and warrants further investigation.35,54 5.ConclusionsThe current study demonstrated significantly greater changes in extracerebral hemodynamics than cerebral hemodynamics during a period of transient hypotension. These findings illustrate the importance of accounting for extracerebral signal contamination with DCS and NIRS measures of cerebral hemodynamics during standard physiological paradigms for evaluating cerebral autoregulation. Specifically, we demonstrated that multilayered analysis applied to DCS data acquired at two source-detector distances and trNIRS substantially reduced the effects of extracerebral signal contamination on cerebral hemodynamic and oxygenation measurements during transient hypotension. The lack of a substantial cerebral hemodynamic response was further confirmed by simultaneously measuring MCAv by TCD and by repeating the LBNP challenge while scalp blood flow was impeded by a head tourniquet, which resulted in a substantially lower blood flow response measured by DCS. DisclosuresThe authors of this manuscript report no relevant financial interests or other potential conflicts of interest to disclose. Code, Data, and Materials AvailabilityThe datasets generated during and/or analyzed during the current study are available from the corresponding author on reasonable request. AcknowledgmentsThis work was funded through grants from the Canadian Institutes of Health Research (Grant No. 130391) and the Natural Sciences and Engineering Research Council of Canada (Grant No. R3592A02002). We thank our participants for their time and effort and J. Kevin Shoemaker for providing access to his lab for data collection. ReferencesH. Ayaz et al.,
“Optical imaging and spectroscopy for the study of the human brain: status report,”
Neurophotonics, 9
(S2), S24001 https://doi.org/10.1117/1.NPh.9.S2.S24001
(2022).
Google Scholar
T. Durduran and A. G. Yodh,
“Diffuse correlation spectroscopy for non-invasive, micro-vascular cerebral blood flow measurement,”
Neuroimage, 85 51
–63 https://doi.org/10.1016/j.neuroimage.2013.06.017 NEIMEF 1053-8119
(2014).
Google Scholar
D. Milej et al.,
“Characterizing dynamic cerebral vascular reactivity using a hybrid system combining time-resolved near-infrared and diffuse correlation spectroscopy,”
Biomed. Opt. Express, 11
(8), 4571 https://doi.org/10.1364/BOE.392113 BOEICL 2156-7085
(2020).
Google Scholar
A. Rajaram et al.,
“Optical monitoring of cerebral perfusion and metabolism in adults during cardiac surgery with cardiopulmonary bypass,”
Biomed. Opt. Express, 11
(10), 5967 https://doi.org/10.1364/BOE.404101 BOEICL 2156-7085
(2020).
Google Scholar
D. Milej et al.,
“Assessing the relationship between the cerebral metabolic rate of oxygen and the oxidation state of cytochrome-c-oxidase,”
Neurophotonics, 9
(3), 035001 https://doi.org/10.1117/1.NPh.9.3.035001
(2022).
Google Scholar
R. C. Mesquita et al.,
“Direct measurement of tissue blood flow and metabolism with diffuse optics,”
Philos. Trans. A. Math. Phys. Eng. Sci., 369
(1955), 4390
–4406 https://doi.org/10.1098/rsta.2011.0232
(2011).
Google Scholar
E. M. Buckley et al.,
“Diffuse correlation spectroscopy for measurement of cerebral blood flow: future prospects,”
Neurophotonics, 1
(1), 011009 https://doi.org/10.1117/1.NPh.1.1.011009
(2014).
Google Scholar
A. Rajaram et al.,
“Perfusion and metabolic neuromonitoring during ventricular taps in infants with post-hemorrhagic ventricular dilatation,”
Brain Sci., 10
(7), 452 https://doi.org/10.3390/brainsci10070452
(2020).
Google Scholar
M. Giovannella et al.,
“BabyLux device: a diffuse optical system integrating diffuse correlation spectroscopy and time-resolved near- infrared spectroscopy for the neuromonitoring of the premature newborn brain,”
Neurophotonics, 6
(2), 025007 https://doi.org/10.1117/1.NPh.6.2.025007
(2019).
Google Scholar
W. B. Baker et al.,
“Continuous non-invasive optical monitoring of cerebral blood flow and oxidative metabolism after acute brain injury,”
J. Cereb. Blood Flow Metab., 39
(8), 1469
–1485 https://doi.org/10.1177/0271678X19846657
(2019).
Google Scholar
S. Y. Lee et al.,
“Quantifying the cerebral hemometabolic response to blood transfusion in pediatric sickle cell disease with diffuse optical spectroscopies,”
Front. Neurol., 13 869117 https://doi.org/10.3389/fneur.2022.869117
(2022).
Google Scholar
M. N. Kim et al.,
“Continuous optical monitoring of cerebral hemodynamics during head-of-bed manipulation in brain-injured adults,”
Neurocrit. Care, 20
(3), 443
–453 https://doi.org/10.1007/s12028-013-9849-7
(2014).
Google Scholar
T. Pham et al.,
“Quantitative measurements of cerebral blood flow with near-infrared spectroscopy,”
Biomed. Opt. Express, 10
(4), 2117 https://doi.org/10.1364/BOE.10.002117 BOEICL 2156-7085
(2019).
Google Scholar
A. B. Parthasarathy et al.,
“Dynamic autoregulation of cerebral blood flow measured non-invasively with fast diffuse correlation spectroscopy,”
J. Cereb. Blood Flow Metab., 38
(2), 230
–240 https://doi.org/10.1177/0271678X17747833
(2018).
Google Scholar
T. Durduran et al.,
“Diffuse optical measurement of blood flow, blood oxygenation, and metabolism in a human brain during sensorimotor cortex activation,”
Opt. Lett., 29
(15), 1766 https://doi.org/10.1364/OL.29.001766 OPLEDP 0146-9592
(2004).
Google Scholar
R. Aaslid, T. M. Markwalder and H. Nornes,
“Noninvasive transcranial Doppler ultrasound recording of flow velocity in basal cerebral arteries,”
J. Neurosurg., 57 769
–774 https://doi.org/10.3171/jns.1982.57.6.0769 JONSAC 0022-3085
(1982).
Google Scholar
P. Sawosz et al.,
“Human skull translucency: post mortem studies,”
Biomed. Opt. Express, 7
(12), 5010 https://doi.org/10.1364/BOE.7.005010 BOEICL 2156-7085
(2016).
Google Scholar
D. Contini et al.,
“Effects of time-gated detection in diffuse optical imaging at short source-detector separation,”
J. Phys. D. Appl. Phys., 48
(4), 045401 https://doi.org/10.1088/0022-3727/48/4/045401 JPAPBE 0022-3727
(2015).
Google Scholar
M. M. Wu et al.,
“Complete head cerebral sensitivity mapping for diffuse correlation spectroscopy using subject-specific magnetic resonance imaging models,”
Biomed. Opt. Express, 13
(3), 1131
–1151 https://doi.org/10.1364/BOE.449046 BOEICL 2156-7085
(2022).
Google Scholar
F. Scholkmann et al.,
“A review on continuous wave functional near-infrared spectroscopy and imaging instrumentation and methodology,”
Neuroimage, 85 6
–27 https://doi.org/10.1016/j.neuroimage.2013.05.004 NEIMEF 1053-8119
(2014).
Google Scholar
T. Sato et al.,
“Reduction of global interference of scalp-hemodynamics in functional near-infrared spectroscopy using short distance probes,”
Neuroimage, 141 120
–132 https://doi.org/10.1016/j.neuroimage.2016.06.054 NEIMEF 1053-8119
(2016).
Google Scholar
Z. Kovacsova et al.,
“Absolute quantification of cerebral tissue oxygen saturation with multidistance broadband NIRS in newborn brain,”
Biomed. Opt. Express, 12
(2), 907 https://doi.org/10.1364/BOE.412088 BOEICL 2156-7085
(2021).
Google Scholar
K. Verdecchia et al.,
“Assessment of a multi-layered diffuse correlation spectroscopy method for monitoring cerebral blood flow in adults,”
Biomed. Opt. Express, 7
(9), 3659 https://doi.org/10.1364/BOE.7.003659 BOEICL 2156-7085
(2016).
Google Scholar
M. Kacprzak et al.,
“Time-resolved imaging of fluorescent inclusions in optically turbid medium - phantom study,”
Opto-Electron. Rev., 18
(1), 37
–47 https://doi.org/10.2478/s11772-009-0027-6 OELREM 1230-3402
(2009).
Google Scholar
G. Blaney et al.,
“Multi-distance frequency-domain optical measurements of coherent cerebral hemodynamics,”
Photonics, 6
(3), 83 https://doi.org/10.3390/photonics6030083
(2019).
Google Scholar
A. Liebert et al.,
“Bed-side assessment of cerebral perfusion in stroke patients based on optical monitoring of a dye bolus by time-resolved diffuse reflectance,”
Neuroimage, 24
(2), 426
–435 https://doi.org/10.1016/j.neuroimage.2004.08.046 NEIMEF 1053-8119
(2005).
Google Scholar
S. A. Carp et al.,
“Combined multi-distance frequency domain and diffuse correlation spectroscopy system with simultaneous data acquisition and real-time analysis,”
Biomed. Opt. Express, 8 3993
–4006 https://doi.org/10.1364/BOE.8.003993 BOEICL 2156-7085
(2017).
Google Scholar
J. Sutin et al.,
“Time-domain diffuse correlation spectroscopy,”
Optica, 3
(9), 1006 https://doi.org/10.1364/OPTICA.3.001006
(2016).
Google Scholar
S. A. Carp, M. B. Robinson and M. A. Franceschini,
“Diffuse correlation spectroscopy: current status and future outlook,”
Neurophotonics, 10
(1), 013509 https://doi.org/10.1117/1.NPh.10.1.013509
(2023).
Google Scholar
J. Selb et al.,
“Sensitivity of near-infrared spectroscopy and diffuse correlation spectroscopy to brain hemodynamics: simulations and experimental findings during hypercapnia,”
Neurophotonics, 1
(1), 015005 https://doi.org/10.1117/1.NPh.1.1.015005
(2014).
Google Scholar
D. Milej et al.,
“Direct assessment of extracerebral signal contamination on optical measurements of cerebral blood flow, oxygenation, and metabolism,”
Neurophotonics, 7
(4), 045002 https://doi.org/10.1117/1.NPh.7.4.045002
(2020).
Google Scholar
D. Milej et al.,
“Incorporating early and late-arriving photons to improve the reconstruction of cerebral hemodynamic responses acquired by time-resolved near-infrared spectroscopy,”
J. Biomed. Opt., 26
(5), 056003 https://doi.org/10.1117/1.JBO.26.5.056003 JBOPFO 1083-3668
(2021).
Google Scholar
J. A. H. R. Claassen et al.,
“Regulation of cerebral blood flow in humans: physiology and clinical implications of autoregulation,”
Physiol. Rev., 101
(4), 1487
–1559 https://doi.org/10.1152/physrev.00022.2020 PHREA7 0031-9333
(2021).
Google Scholar
N. Goswami et al.,
“Lower body negative pressure: physiological effects, applications, and implementation,”
Physiol. Rev., 99
(1), 807
–851 https://doi.org/10.1152/physrev.00006.2018 PHREA7 0031-9333
(2019).
Google Scholar
W. B. Baker et al.,
“Pressure modulation algorithm to separate cerebral hemodynamic signals from extracerebral artifacts,”
Neurophotonics, 2
(3), 035004 https://doi.org/10.1117/1.NPh.2.3.035004
(2015).
Google Scholar
D. Milej et al.,
“Quantification of cerebral blood flow in adults by contrast-enhanced near-infrared spectroscopy: validation against MRI,”
J. Cereb. Blood Flow Metab., 40
(8), 1672
–1684 https://doi.org/10.1177/0271678X19872564
(2020).
Google Scholar
M. Khalid et al.,
“Development of a stand-alone DCS system for monitoring absolute cerebral blood flow,”
Biomed. Opt. Express, 10
(9), 4607 https://doi.org/10.1364/BOE.10.004607 BOEICL 2156-7085
(2019).
Google Scholar
M. Kewin et al.,
“Evaluation of hyperspectral NIRS for quantitative measurements of tissue oxygen saturation by comparison to time-resolved NIRS,”
Biomed. Opt. Express, 10
(9), 4789 https://doi.org/10.1364/BOE.10.004789 BOEICL 2156-7085
(2019).
Google Scholar
A. Abdalmalak et al.,
“Using fMRI to investigate the potential cause of inverse oxygenation reported in fNIRS studies of motor imagery,”
Neurosci. Lett., 714 134607 https://doi.org/10.1016/j.neulet.2019.134607 NELED5 0304-3940
(2020).
Google Scholar
H. Liu et al.,
“Influence of blood vessels on the measurement of hemoglobin oxygenation as determined by time-resolved reflectance spectroscopy,”
Med. Phys., 22
(8), 1209
–1217 https://doi.org/10.1118/1.597520 MPHYA6 0094-2405
(1995).
Google Scholar
A. Liebert et al.,
“Evaluation of optical properties of highly scattering media by moments of distributions of times of flight of photons,”
Appl. Opt., 42
(28), 5785 https://doi.org/10.1364/AO.42.005785 APOPAI 0003-6935
(2003).
Google Scholar
D. Milej et al.,
“Optimization of the method for assessment of brain perfusion in humans using contrast-enhanced reflectometry: multidistance time-resolved measurements,”
J. Biomed. Opt., 20
(10), 106013 https://doi.org/10.1117/1.JBO.20.10.106013 JBOPFO 1083-3668
(2015).
Google Scholar
D. Milej et al.,
“Time-resolved subtraction method for measuring optical properties of turbid media,”
Appl. Opt., 55
(7), 1507 https://doi.org/10.1364/AO.55.001507 APOPAI 0003-6935
(2016).
Google Scholar
A. Abdalmalak et al.,
“Assessing the feasibility of time-resolved fNIRS to detect brain activity during motor imagery,”
Proc. SPIE, 9690 969002 https://doi.org/10.1117/12.2209587 PSISDG 0277-786X
(2016).
Google Scholar
R. Re et al.,
“Multi-channel medical device for time domain functional near infrared spectroscopy based on wavelength space multiplexing,”
Biomed. Opt. Express, 4
(10), 2231
–2246 https://doi.org/10.1364/BOE.4.002231 BOEICL 2156-7085
(2013).
Google Scholar
K. Verdecchia et al.,
“Assessment of the best flow model to characterize diffuse correlation spectroscopy data acquired directly on the brain,”
Biomed. Opt. Express, 6
(11), 4288 https://doi.org/10.1364/BOE.6.004288 BOEICL 2156-7085
(2015).
Google Scholar
G. Li, J. T. Bronk and P. J. Kelly,
“Canine bone blood flow estimated with microspheres,”
J. Orthop. Res., 53 7
–11 https://doi.org/10.1002/jor.1100070109 JOREDR 0736-0266
(1989).
Google Scholar
G. E. Strangman, Z. Li and Q. Zhang,
“Depth sensitivity and source-detector separations for near infrared spectroscopy based on the Colin27 brain template,”
PLoS One, 8
(8), e66319 https://doi.org/10.1371/journal.pone.0066319 POLNCL 1932-6203
(2013).
Google Scholar
J. Duffin, D. J. Mikulis and J. A. Fisher,
“Control of cerebral blood flow by blood gases,”
Front. Physiol., 12 640075 https://doi.org/10.3389/fphys.2021.640075 FROPBK 0301-536X
(2021).
Google Scholar
V. L. Kay and C. A. Rickards,
“The role of cerebral oxygenation and regional cerebral blood flow on tolerance to central hypovolemia,”
Am. J. Physiol. - Regul. Integr. Comp. Physiol., 310 R375
–R383 https://doi.org/10.1152/ajpregu.00367.2015
(2016).
Google Scholar
S. Houtman et al.,
“Changes in cerebral oxygenation and blood flow during LBNP in spinal cord-injured individuals,”
J. Appl. Physiol., 91 2199
–2204 https://doi.org/10.1152/jappl.2001.91.5.2199
(2001).
Google Scholar
J. Hisdal et al.,
“Associations between changes in precerebral blood flow and cerebral oximetry in the lower body negative pressure model of hypovolemia in healthy volunteers,”
PLoS One, 14
(6), e0219154 https://doi.org/10.1371/journal.pone.0219154 POLNCL 1932-6203
(2019).
Google Scholar
H. Zhao and E. M. Buckley,
“Influence of source–detector separation on diffuse correlation spectroscopy measurements of cerebral blood flow with a multilayered analytical model,”
Neurophotonics, 9
(3), 035002 https://doi.org/10.1117/1.NPh.9.3.035002
(2022).
Google Scholar
J. Wu et al.,
“Two-layer analytical model for estimation of layer thickness and flow using Diffuse Correlation Spectroscopy,”
PLoS One, 17
(9), 1
–20 https://doi.org/10.1371/journal.pone.0274258 POLNCL 1932-6203
(2022).
Google Scholar
BiographyLeena N. Shoemaker is a postdoctoral associate at Western University in London, Canada. She received her PhD in cerebrovascular and exercise physiology from Otago University in Dunedin, New Zealand, in 2020. Her current research focuses on utilizing a combination of non-invasive imaging methods to elucidate the vascular mechanics of the cerebral micro- and macro-vasculature. She is particularly interested in the role of exercise in dampening age-related declines in cerebrovascular and cognitive function. Daniel Milej is a research associate at Lawson Health Research Institute in London, Canada. He received his PhD in biomedical engineering from the Nalecz Institute of Biocybernetics and Biomedical Engineering, Polish Academy of Sciences, in 2014. His current research focuses on designing, developing, and implementing sophisticated optoelectronic medical and clinical instrumentation. He is particularly interested in brain imaging using time-domain diffuse optics and diffuse correlation spectroscopy. Jigneshkumar Mistry is an MSc candidate in the Department of Medical Biophysics at Western University. He received his MTech in biomedical engineering from Indian Institute of Technology, Chennai, in 2021. His current research focuses on assessing cerebral microvasculature using optical devices. He is specifically interested in measuring critical closing pressure from the cerebral microvasculature using diffuse correlation spectroscopy and removing extracerebral contamination from it. Keith St. Lawrence is a professor in the Department of Medical Biophysics at Western University. His research focuses on developing quantitative methods of imaging cerebral blood flow and energy metabolism. His research encompasses a number of modalities, including diffuse optics, functional near-infrared spectroscopy, magnetic resonance imaging, and positron emission tomography. |
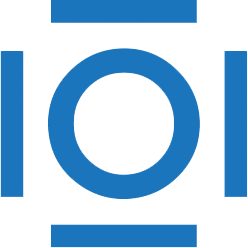
CITATIONS
Cited by 3 scholarly publications.
Blood circulation
Near infrared spectroscopy
Brain
Data modeling
Spectroscopy
Hemodynamics
Contamination