|
ACCESS THE FULL ARTICLE
No SPIE Account? Create one
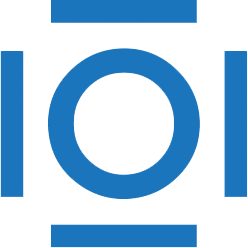
CITATIONS
Cited by 9 scholarly publications.
Wavelets
Principal component analysis
Calibration
Spectral calibration
Data compression
Hyperspectral imaging
Computing systems