|
ACCESS THE FULL ARTICLE
No SPIE Account? Create one
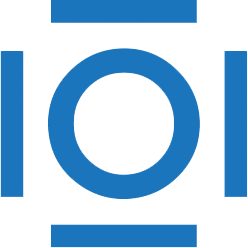
CITATIONS
Cited by 12 scholarly publications.
Neural networks
Damage detection
Neurons
Evolutionary algorithms
Pattern recognition
Feature extraction
Calibration